Keynotes
1st Keynote Speaker
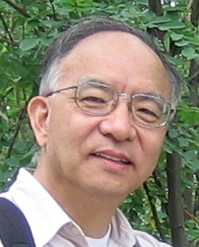
Abstract: The real-world big data are largely dynamic, interconnected, and unstructured texts. It is important to transform such massive unstructured data into structured knowledge. Many researchers rely on labor-intensive labeling and curation to extract knowledge from text data. Such approaches, however, are not scalable. We vision that massive text data itself may disclose a large body of hidden structures and knowledge. Equipped with pretrained language models and machine learning methods, it is promising to transform unstructured data into structured knowledge. In this talk, we overview a set of weakly supervised machine learning methods developed recently in our group for such an exploration, including joint spherical text embedding, discriminative topic mining, taxonomy construction, text classification, and taxonomy-guided text analysis. We show that weakly supervised approach could be promising at transforming massive text data into structured knowledge.
Bio: Jiawei Han is Michael Aiken Chair Professor in the Department of Computer Science, University of Illinois at Urbana-Champaign. He received ACM SIGKDD Innovation Award (2004), IEEE Computer Society Technical Achievement Award (2005), IEEE Computer Society W. Wallace McDowell Award (2009), and Japan's Funai Achievement Award (2018). He is Fellow of ACM and Fellow of IEEE and served as the Director of Information Network Academic Research Center (INARC) (2009-2016) supported by the Network Science-Collaborative Technology Alliance (NS-CTA) program of U.S. Army Research Lab and co-Director of KnowEnG, a Center of Excellence in Big Data Computing (2014-2019), funded by NIH Big Data to Knowledge (BD2K) Initiative. Currently, he is serving on the executive committees of two NSF funded research centers: MMLI (Molecular Make Research Institute)—one of NSF funded national AI centers since 2020 and I-Guide—The National Science Foundation (NSF) Institute for Geospatial Understanding through an Integrative Discovery Environment (I-GUIDE) since 2021.
2nd Keynote Speaker
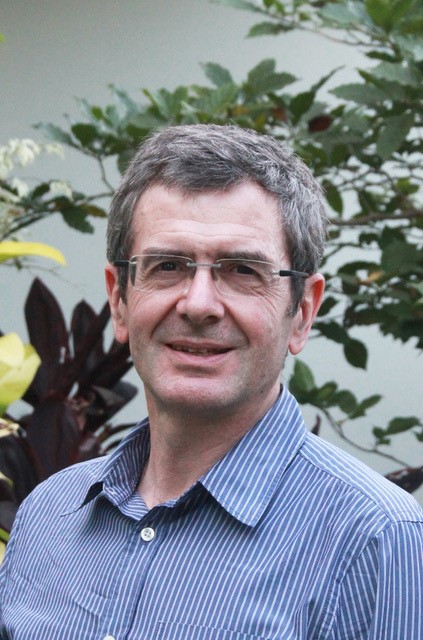
Prof. Thierry Denoeux
Professor
Université de Technologie de Compiègne
Title: Random Fuzzy Sets and Belief Functions: Application to Machine Learning
Abstract: The theory of belief functions is a powerful formalism for uncertain reasoning, with many successful applications to knowledge representation, information fusion, and machine learning., Until now, however, most applications have been limited to problems (such as classification) in which the variables of interest take values in finite domains. Although belief functions can, in theory, be defined in infinite spaces, we lacked practical representations allowing us to manipulate and combine such belief functions. In this talk, I show that the theory of epistemic random fuzzy sets, an extension of Possibility and Dempster-Shafer theories, provides an appropriate framework for evidential reasoning in general spaces. In particular, I introduce Gaussian random fuzzy numbers and vectors, which generalize both Gaussian random variables and Gaussian possibility distributions. As an illustration, I show an application of this new formalism to nonlinear regression.
Related reference:
T. Denoeux, Reasoning with fuzzy and uncertain evidence using epistemic random fuzzy sets: general framework and practical models. Preprint arXiv:2202.08081, https://doi.org/10.48550/arXiv.2202.08081Bio: Thierry Denoeux is a Full Professor with the Department of Information Processing Engineering at the University of Compiègne, France, and a senior member of the French Academic Institute (Institut Universitaire de France). His research interests concern reasoning and decision-making under uncertainty and, more generally, the management of uncertainty in intelligent systems. His main contributions are in the theory of belief functions with applications to statistical inference, pattern recognition, machine learning and information fusion. He has published more than 300 papers in this area. He is the Editor-in-Chief of the International Journal of Approximate Reasoning, and an Associate Editor of several journals including Fuzzy Sets and Systems and International Journal on Uncertainty, Fuzziness and Knowledge-Based Systems.
3rd Keynote Speaker
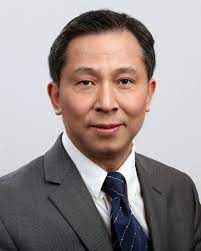
Prof. Hui Xiong
Chair Professor, Fellow of AAAS and IEEE
the Hong Kong University of Science and Technology Guangzhou, China
Title: Talent Analytics: Prospects and Opportunities
Abstract: The big data trend has made its way to human resource management. Indeed, the availability of large-scale human resource (HR) data provide unparalleled opportunities for business leaders to understand talent behaviors and generate useful talent knowledge, which in turn deliver intelligence for real-time decision making and effective people management at work. In this talk, we introduce the powerful set of innovative Artificial Intelligence (AI) techniques developed for intelligent human resource management, such as recruiting, performance evaluation, talent retention, talent development, job matching, team management, leadership development, and organization culture analysis. In addition, we will also demonstrate how the results of talent analytics can be used for other business applications, such as market trend analysis and financial investment.
Bio: Dr. Hui Xiong is currently a Chair Professor at the Hong Kong University of Science and Technology Guangzhou, China. Dr. Xiong’s research interests include data mining, mobile computing, and their applications in business. Dr. Xiong received his PhD in Computer Science from University of Minnesota, USA. He has served regularly on the organization and program committees of numerous conferences, including as a Program Co-Chair of the Industrial and Government Track for the 18th ACM SIGKDD International Conference on Knowledge Discovery and Data Mining (KDD), a Program Co-Chair for the IEEE 2013 International Conference on Data Mining (ICDM), a General Co-Chair for the 2015 IEEE International Conference on Data Mining (ICDM), and a Program Co-Chair of the Research Track for the 2018 ACM SIGKDD International Conference on Knowledge Discovery and Data Mining. He received the 2021 AAAI Best Paper Award and the 2011 IEEE ICDM Best Research Paper award. For his outstanding contributions to data mining and mobile computing, he was elected an AAAS Fellow and an IEEE Fellow in 2020.